What is the impact of Generative AI and related technologies on research photography? Will it undermine the need to collect original photos? Or extend their impact?
Despite being a niche topic, research photography makes an interesting case study because of its relatively high return on investment compared to other data types and the broad impact from emerging technologies.
—
Previous articles in this series covered first principles, explored the impact of Generative AIs on the evolution of understanding, introduced the concept of organisational data metabolism as a way of thinking about an organisation’s propensity to effectively ingest different types of data, before unpacking the foundational value of original photography. These are all topics that I’m researching for my next book on human and organisational sensemaking, a follow up to The Field Study Handbook.
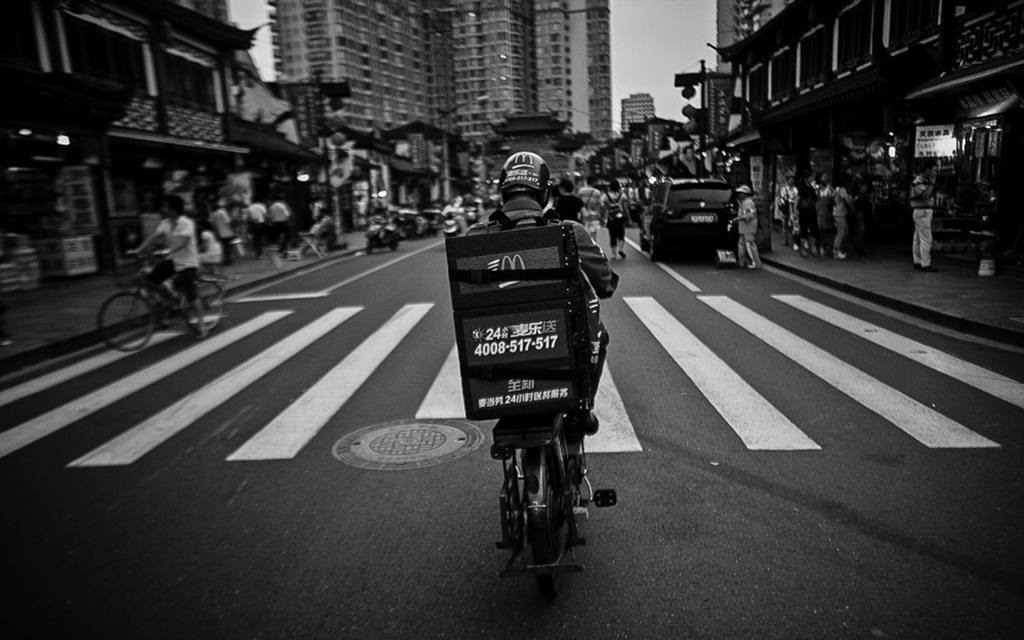
How asks evolve
Research photography is one of many qualitative data-gathering methods. To understand how it is used today, we can consider the kinds of qualitative research projects that are commissioned by organisations.
As qualitative research teams demonstrate their value (or otherwise) to their clients, demands on their time typically evolve from tactical requests such as “Help us fix this” and “Help us make this” to more strategic ones—the most common being “What should we make?” with insights and recommendations grounded in user needs and behaviours. The following framework was originally proposed by my manager at Nokia Research Panu Korhonen, which I’ve extended to encompass Studio D client asks from the past decade.
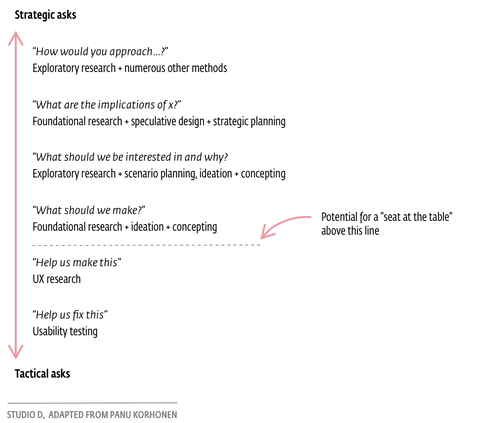
FIGURE 1: The evolution of client asks
A milestone for the research team occurs when requests shift from tactical requests to more open-ended, strategic questions. Whether this leads to a “seat at the decision-making table” depends on many factors such as demonstrating enduring value, political nous, and integrating well with other organisational functions. The more open-ended the starting questions, the further upstream in decision making process the team moves, the greater the number of organisational functions that it potentially affects.
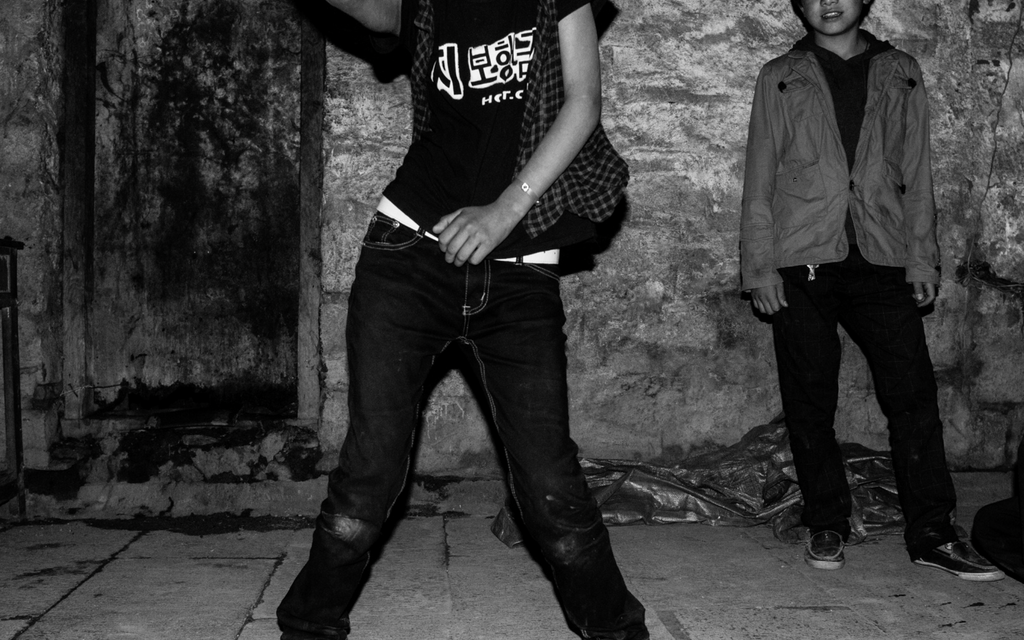
Starting assumptions
Before we move onto future scenarios I've seeded a few assumptions drawn from a reflection of why our practice norms settled where they are today, combined with extrapolations of current trends. Of course these are open to debate and many other assumptions can and should be considered.
Qualitative sensemaking assumptions
E.g. historically, well run qualitative research has provided an nuanced understanding of “why” people behave as they do, whilst well run quantitative data delivers on the “what” and “how”. AI seems well poised to tackle “what if” questions with some important caveats around the black-box nature of their training data and inherent, difficult-or-impossible to mitigate biases. My assumption is that commissions for field research will gradually decline (in relation to other research methodologies) as more of our daily lives shift-to and are queryable online—but that organisations will continue to value the nuanced “understanding why” perspective drawn from in-field data.
Cultural assumptions
E.g. mainstream access to vastly more content of which the veracity is more difficult to gauge. However, in certain scenarios, a higher tolerance for errors will be considered acceptable trade-offs against convenience and speed (the impact of this on what products are released on the market, and the speed of trail and error in the market place is worthy of a separate article).
Our community values information accuracy but, as practitioners who study the human condition we also recognise that person-to-person transmission of information is inherently prone to distortions from intent and fallibilities in recall. I posit that we’ve always lived in a disinformation landscape, and the current turmoil is from the shift to new gatekeepers of the “truth”, the increased scale and the speed at which disinformation is disseminated.
Organisational assumptions
E.g. the speed at which new technologies are adopted is accelerating.
Whilst deploying new technologies organisation-wide is non-trivial it is influenced by employee’s practices outside the workplace. This influence is especially true of practices drawn from social media adoption since a deep understanding of these platforms—and the new technologies, features, and assumptions they deploy—are gained from hands-on use and abuse. Motivations for using social media varies across generations as a reflection of the the motivations at different life-stages (Armstrong, 2016, Chipchase, 2021). This creates a “generational mindset shift” of employees who are “natives” versus “non-natives”, and as more senior staff make way for new employees it becomes easier to challenge deeply held assumptions of best practice in a given domain.
Technological assumptions
E.g. that Generative AI can produce photos, video, audio, text, code and other content types; more “localised” AI models based on country-, regional-, and political-leanings, religious belief systems; more refined content fingerprinting and content tracking; more evolved augmented reality (plus a few niche virtual reality use cases); plus widespread consumer-grade tools for mapping spaces using Lidar and related technologies.
There will continue to be inherent unaddressed biases in the generative AI models, and its an open question what future level of transparency they will provide to their training data and processes, mandated by regulation or by societal pressure.
Regulatory assumptions
The regulatory environment for AI is still nascent, although based on past stances the relative emphasis I’ve assumed are:
- US: emphasis on business opportunities, then socetial wellbeing,
- China: emphasis on protecting the state, but otherwise pragmatically business first,
- European Union: emphasis on quality of life, then business opportunities.
The European Parliament’s AI Act (2024), currently going through ratification includes provisions relevant to this article including: the labelling of AI generated and manipulated imagery; that data sets (include those built on generating images) are “relevant, representative, free of errors and complete”, taking into account the intended purpose (Edwards, 2024); and limitations e.g. on police and some other uses of real-time facial recognition systems.
Each country/trading block is juggling a different set of stakeholders and threats, and other approaches to regulation will emerge. India, Pakistan and Japan make strong alternative case studies to consider because of the issues they are facing, attitudes to privacy, and the role of the state.
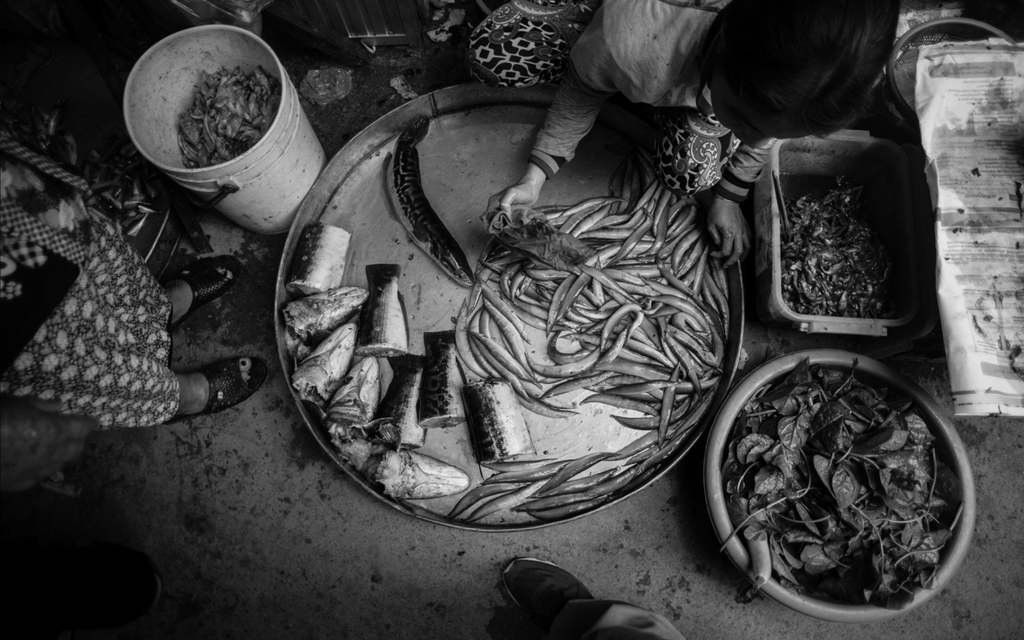
Current research photography workflow
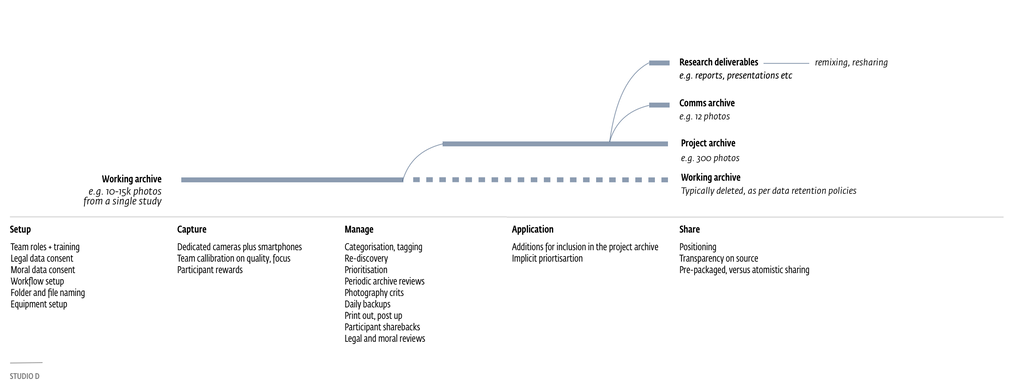
FIGURE 2. Current research photography workflow
In the current research photography workflow we assign one person on the in-field team as “data manager” whose role includes the oversight and delegation of photography management tasks. This role typically takes about 10-15% of their time, in addition to other project duties. For example, each research team member interprets the value of a photo differently, so the data manager is responsible for ensuring each member of the team—both locals and international staff—systemically reviews, cleans up, tags, and prioritises photos that their interview team has collected.
Legal and moral consent, review
We require our photo archive to only contain photos from interviews and personal spaces that we have participant's permission to collect. The full circle photographic data collection process goes some way to addressing this issue, and include two distinct review processes for photos that are worth highlighting:
- Legal review. This is relatively straightforward—one either has data consent that gives permission for the organisation to have the photos and puts boundaries on their use, or one doesn’t. Photos without data consent either require a follow up request to the participant for consent, or are purged from the archive.
- Moral review. For photos that we have legal consent, we also conduct multiple rounds of moral reviews that essentially poses the questions: “How would this person feel if this image of them and the contexts in which they were photographed were used by organisation?”; “What are the risks to the participant if our organisation has these photos?”; and “What steps can be take to remove these risks?”. Sometimes the moral review results in compelling photos being deleted, at other times personally identifiable information (PII) is filtered out, and sometimes we present compelling photos in an abstracted form i.e. through an illustration.
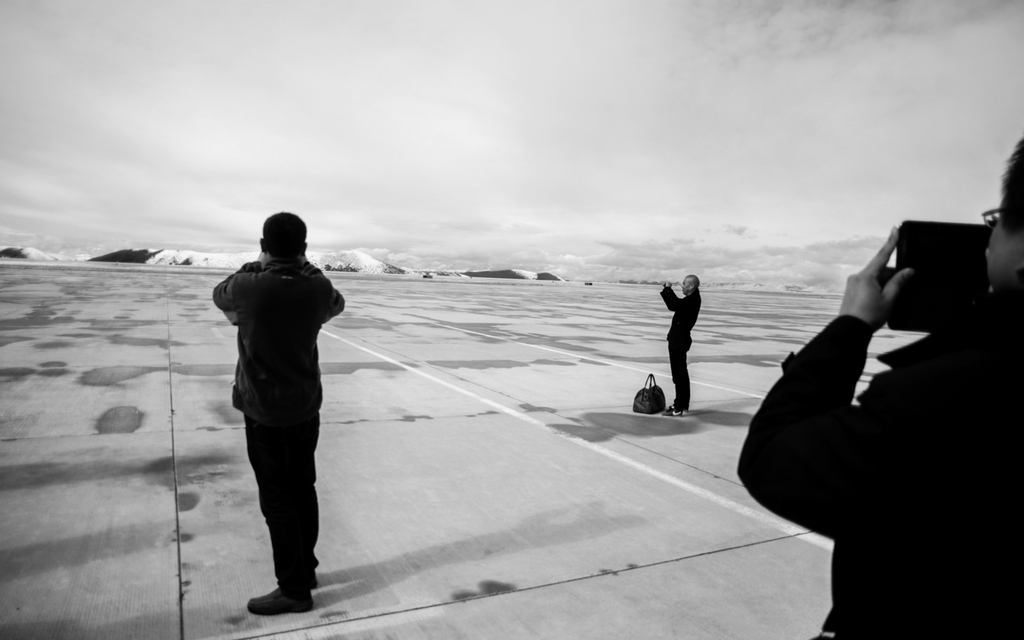
Seven emergent scenarios
Based on our our prior assumptions and existing workflow, we can imagine possible scenarios and propose a new workflow. These examples are selected from over thirty that flowed naturally from writing this article—I’m anticipating many more to be generated from attendees of the Photography in the Paradigm Shift Masterclass that I’m running in May.
Scenario 1: Adding a “localised” layer to existing generative models
Generative AI models can be trained on hundreds of millions of photos and other data, so the number of photos collected on a single study—10-15k on a typical Studio D project—is a drop in the ocean. That said, my hunch is that this is still sufficient to train or tune a “localised” generative AI that improves the relevance and value of the outputs of its more general underlying model. Once a localised generative model is built for a specific domain—such as an industry, business unit, or domain they can be reused and periodically updated.
This approach doesn’t address the biases inherent in the underlying model, but may mitigate outputs be more representative and relevant. Of course a qualitative research study doesn't just generate photos, but can include interview transcripts, video, audio, sometimes also analytics and other contextual data—all of which can be used to refine the localised model.

FIGURE 3. Generalised and localised AI models
How long is a localised model likely to remain relevant?
One way to consider this question is through the Shearing Layers framework proposed by British architect Frank Duffy. It recognises that certain things like the structure of a building changes slowly if at all, whereas other layers such as building services, the layout of a space, or objects that populate a space will change at a faster pace. Adopting this framework, our localised generative model will contain some layers that are effectively out-of-date within a few years if someone wanted to generate a new, current situation, whilst other layers will likely outlive the organisation that deploys the model.
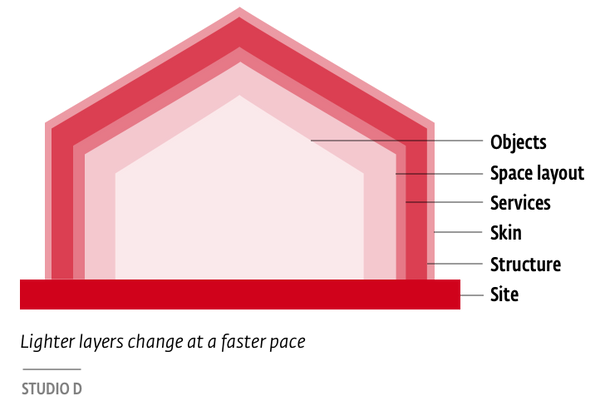
FIGURE 4. Frank Duffy's Shearing Layers
Knowing that photos and associated data will be used to train AI models—with all the responsibility this entails—will affect the range and type of data collected, and how it is collected.
Scenario 2: Applying a localised Generative AI
How might a localised generative AI model be applied in an organisation?
Whilst the veracity of photos forms the current basis of the researcher/audience social contract, the norms of downstream organisational teams support ways to to apply the localised model for showing e.g. prototypes in new contexts, scenario planning, provide stimuli for marketing and communications.
Scenario 3: Abstraction and in-house styles
If photographic-style Generative AI imagery retain all the aesthetic values of our localised training archive then the results are inherently problematic—presented with the look and feel of an authentic photo they break the research/audience social contract. Watermarking and captioning outputs as AI-generated are clunky solutions that in many cases depreciate the value or human-collected data and deliverables.
FIGURE 5. The creator/audience social contract
The social contract between a creator and their audience is built on a shared understanding and adherence to the norms of their domain. For the research community this includes data consent, PII clean up, minimal manipulation, and transparency on where the dat was sourced. Breaking the creator/audience social contract without good reason i.e. delivering sufficient value, results undermines trust not just in a single piece of content, but potentially the whole research approach. There’s a lot at stake.
To maintain trust I anticipate organisations will settle on the following generative applications for research photography:
- Obscurification For example, consistently removing PII from a scene, or in situations where the presentation of the original context is paramount, consistently replacing a real participant’s face with an artificially generated one to protect their identity.
-
Generative Style Guides In the same way that organisation's have in-house guidelines for an their look-and-feel identity that applies to their website, documents, apps, and advertising campaigns, organisations will develop in-house style guides for AI generated imagery that reflects their organisational values and aesthetic. A major benefit of this is to signal that “images in this style” are artificially generated. There can be multiple overlapping or distinct Generative Style Guides across an organisation—such as one for research outputs, product, scenario planning, communications—generally reflecting the needs and norms of each professional community.
- Abstraction Signalling that an image is AI generated for example to protect identities, or to make the outputs representative of a broader range of people. Using Scott McCloud’s illustrations from Understanding Comics (1993), and starting from a project photo archive we can dial the level of abstraction of Generative AI outputs to be representative of between tens or billions of people, depending on what is required.
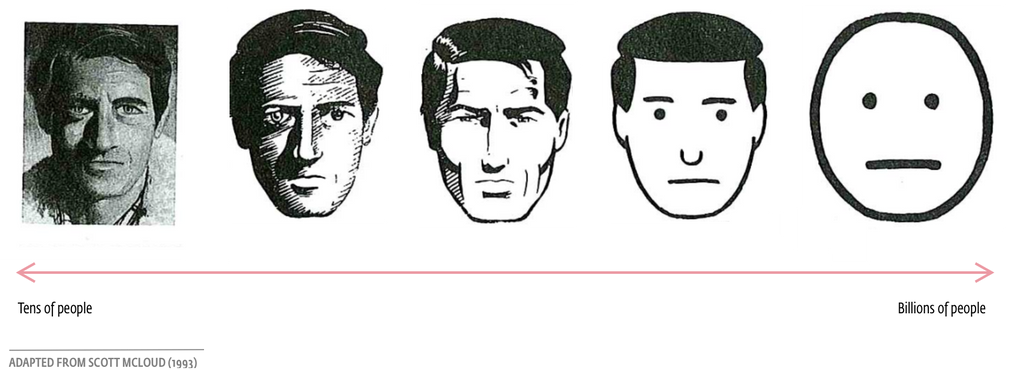
FIGURE 6: Levels of abstraction
There are strong legal and moral drivers for adopting in-house styles and abstraction for Generative AI outputs, namely:
- the social contract (and sometimes legal requirement) to indicate how an photo or photography-style image was captured or generated, and,
- the moral and legal requirements covered by the data consent.
Scenario 5: Photo and Photo-like Classification System
To better maintain the integrity of authentic photos being presented alongside AI generated photo-like imagery, a classification system is also required to communicate, amongst other things:
- the source/s of photos and photo-like images, including insight into training data and other contextual information,
- the level of manipulation e.g. retouching or filters (in widespread use today), inpainting or outpainting.
- for Generative AI outputs, the list of prompts used to create the image, and,
- the style-sheets and abstractions that were applied.
I anticipate public domain classification systems—analogous to a combination of EXIF meta-data standards, Creative Commons for copyright, and the Chicago Manual of Style for written content. Some organisations will develop their own classification systems that reflect their localised needs.
Scenario 6: Data consent & participant rewards
Current data consent and participant reward protocols will need to be updated to account for wider scenarios of data use, new forms of value creation, and the longevity over which data will effectively live on in an organisation.
Scenario 7: Mapping then generating spaces
That consumer grade Lidar and similar technologies enable researchers to generate 3D models of spaces with minimal disruption to existing research protocols (there is speculation that OpenAI's generative video-from-text tool Sora was trained on data that includes game engines). These 3D models can then be applied to more accurate, nuanced outputs, including high resolution photo-like video and photo-like imagery.
Reflecting on the scenarios
If, like me, your initial reaction is to recoil at some of these scenarios and how they undermine the hard-fought norms of today’s research community, consider how we have already accepted degrees of photo manipulation, abstraction and support reinterpretation within our practice—such as the use of archetypes, or the use of photos without captions.
Update: Tom Hoy pointed me to Retrieval Augmented Generation (RAG), that takes an analogous approach to re-contextualising outputs.
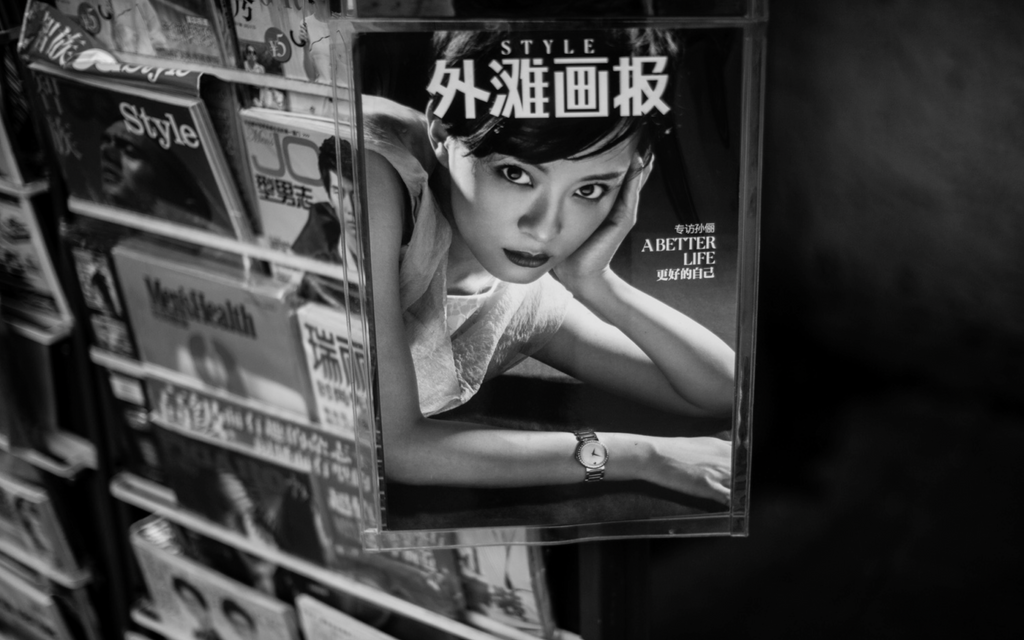
Updating our photography goals
Today, when we conduct field research our broad goal with photography is “to build a representative, accessible and versatile project archive of high quality, appropriately sourced research photography”.
FIGURE 7. The updated goal of project photography
In the medium term we might want to adapt this goal to include:
- “defensible”, i.e. it demonstrates the rigour applied to meeting thresholds of “representation” and “appropriate sourcing”, since the data (photos, video, 3D models) collected can be applied by a wider variety of situations. Being able to explain decisions is not new, but the legal implications from regulation will likely heighten the need for defensibility,
- “and other assets”, e.g. interview transcripts, or the collection of 3D models of the spaces that our research participants inhabit;
Updating the photography & generative workflow
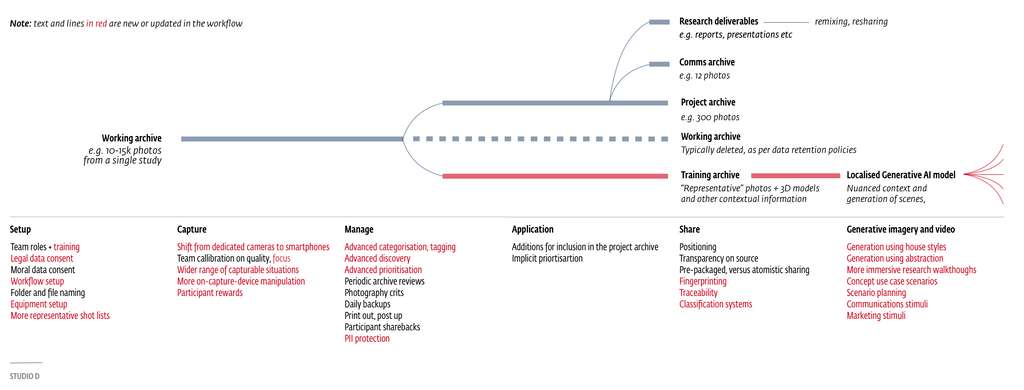
FIGURE 8. The updated photography and generative workflow
Closing thoughts
Exposing these ideas to a wider audience has been a healthy forcing function for me to crystallise ideas for my next book on human and organisational sensemaking, and connect with other members of this community facing similar issues.
Some of the scenarios I’ve outlined here may come to pass whilst other’s will not. However I believe the underlying rationale for proposing them will continue to be relevant and provides plenty of scope to explore alternatives.
It seems like every professional domain is undergoing a paradigm shift as they seek to understand the roles that can humans play in an age of AI. The qualitative research community has valuable skills and perspectives to contribute and better navigate the changes ahead—given that we revel in complexity and in extrapolating second order effects.
If the qualitative research community wants to continue to be asked to identify and solve strategic questions, we need to think strategically about the opportunities and threats to our own practice, re-evaluating why we have adopted our existing norms—and propose new ways forward. The benefits of our contribution go well beyond our working horizons, affect how others domains can frame what all peoples should expect from emerging technologies.
//
If you enjoyed this article, I'd appreciate you putting the word out.
Finally, if your interest is sufficiently piqued in this subject please join the Photography in the Paradigm Shift Masterclass where we’ll cover the topics covered in this series in more depth.
Foot Notes
On motivational drivers for use of social media platforms… this 12-life stage framework (Armstrong, 2016) is a good starting point, if one recognises variations in this model due to cultural context and personality differences. For example, forming an identity during adolescence, finding one’s tribe in early adulthood, or maintaining a work network during mid-life.
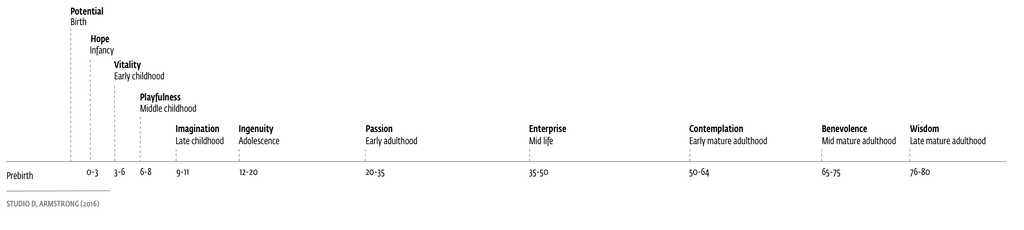
FIGURE 9. Attributes driving motivational shift across life stages
On organisational assumptions... “generational cycles” of practitioner mindsets seem be contracting, which can have a profound impact on “organisational wisdom”, and likely reinforces the importance of defining values and purpose to maintain organisational stability and coherence.
On systematically understanding sensemaking approaches and outcomes… there’s a dearth of case studies and peer reviewed papers, so I’m leaning heavily on conversations and experiences over the course of my career.
On the power of abstraction… one of the reasons why I commissioned Lee John Phillips illustrations in The Field Study Handbook rather than print the original photos that they were based on, is that the photos represent my experiences, whereas illustrations provide latitude for readers to imagine themselves living those experiences.
On Frank Duffy's Shearing Layers... the concept was expanded upon by Stewart Brand who proposed the more widely referenced Pace Layers concept.
On the creator/audience social contract... In selected circumstances the creator can subvert the norms of a domain or genre, and this can still be considered acceptable by the audience if it later demonstrates additional value from that subversion. A mainstream equivalent might be a noir detective TV series that follows the expectations of its genre, but that shifts to an intrepretive dance for its closing scenes
On legal and moral photo archive reviews... a hat-tip to the former Head of Legal at frog—Cyrus Ipaktchi who proposed framing all data as potentially toxic—and therefore capable of contaminating an organisation—something that has guided my thinking about suitable data-handling processes over the years.
On the book writing process... I've been working on this for two years thus far, and it feels like another ~six years will be required to wrap my head around the many domains that human and organisational sensemaking touches upon.
References
- The Human Odyssey: Navigating the Twelve Stages of Life (2016) Thomas Armstrong.
- Life Stage Social Media Adoption (2021) Jan Chipchase, unpublished.
- EU AI Act: first regulation on artificial intelligence (2023). European Parliament
- The EU AI Act: a summary of its significance and scope (2024) Lilian Edwards, Newcastle University.
- China vs US Approaches to AI Governance (2023). Adam Lu, The Diplomat
- Understanding Comics (1993), Scott McCloud.
- Evaluating the accuracy and quality of an iPad Pro's built-in lidar for 3D indoor mapping, (2023) Tee-Ann Teo & Chen-Chia Yang, Developments in the Built Environment, Volume 14.
Original photos by author:
- Customised rickshaw mud flaps, Hyderabad, India.
- Takeout, Pudong, China.
- K-pop advocates, Lhasa, Tibet Autonomous Region, China.
- Lashio wet market, Myanmar.
- Disembarking passengers, Daocheng Yading Airport, China.
- Media stand, Shanghai, China.
- Camp Nou, Real Madrid versus Barcelona, Spain.